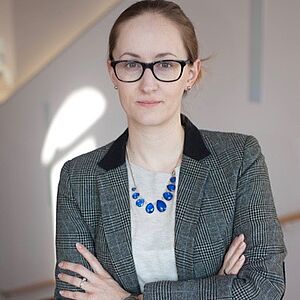
Axes de recherche
Feature Distribution Learning
We developed a new method called Feature Distribution Learning to study representations of visual ensembles and our results revealed that observer’s representations of visual features are far more detailed than previous studies of ensemble perception have suggested. Ensemble perception emphasizes on summary statistics, i.e., mean and variance. However, observers can represent surprisingly complex distribution shapes such as whether a representation is Gaussian, uniform or bimodal. Our method proved to be an important implicit way of assessing how observers represent regularities in their environments.Seminal work on developing and implementing this new approach was done by Dr. Andrey Chetverikov. It has been applied to study various visual features such as color, orientation, a combination of visual features (orientation and color simultaneously) and recently also for shapes or contours.
Temporal Integration of Probabilistic Information
Humans are surprisingly good at learning the characteristics of their visual environment. Recent studies have revealed that the visual system encodes detailed information about the distractor distribution characteristics during a visual search task (see Project on Feature Distribution Learning). Search times are determined by the frequency of distractor features over consecutive search trials. In this study, we found that a similar learning of frequencies is also visible for single targets in a visual search task. This is remarkable given that observers are only presented with a single exemplar of the distribution on each trial. Results from our experiments confirm the existence of an internal representation of target feature distributions, but also that the visual system integrates probability distributions of features over surprisingly long trial sequences.
Attractive and Repulsive Biases In Perception
In this project we investigate if the visual system utilizes multiple sources of information to optimize perception of visual ensembles such as when we search for targets among distractors. This work is part of the PhD thesis of Mohsen Rafiei advised by Prof. Árni Kristjánsson, Dr. Andrey Chetverikov and myself. Humans have remarkable abilities to construct a stable visual world from continuously changing input. There is increasing evidence that our momentary visual input blends with previous input to preserve perceptual continuity, a phenomenon called serial dependence. However, little is known about the role of ignored stimuli in creating this continuity. This is important since while some input is selected for processing, other input must be actively ignored for efficient selection of the task-relevant stimuli. We asked whether attended targets and actively ignored distractor stimuli in an odd-one-out search task would bias observers’ perception differently.
Results from a series of studies show that at least two opposite biases influence current perception: A positive bias caused by serial dependence pulls perception of the target toward the previous target features, while a negative bias induced by the to-be-ignored distractor features pushes perception of the target away from the distractor distribution. Our results suggest that to-be-ignored items produce a perceptual bias that acts in parallel with other biases induced by attended items to optimize perception.
Additionally, I am currently investigating the role of uncertainty on both, the attractive and repulsive bias and how it affects visual confidence.