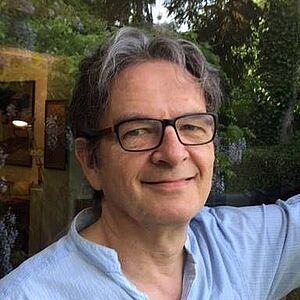
Philippe Devienne
Research Focus
Spiking neuron networks for neuromorphic computing and bioacoustic
Nanospike project :
The research aims to discover new paradigms for temporal pattern recognition. A Spiking Neuron Network (SNN) is the best suited model to do so since the information it processes can be injected on line, in the form of spikes, as input to the network. Modeling asynchronous processing in the brain, information in SNNs is mainly coded by the precise timing of spikes, at all levels: input, internal processing, output. The present learning paradigms for SNNs do not fully harness the temporal feature of data yet. Data are usually received as real number matrices and then translated into spike trains, more or less clumsily. Although SNNs carry a high computing potential, well identified by many scientists, the power of SNNs has not yet been fully exploited for pattern recognition and for processing temporal data, e.g. speech, video. The spatio-temporal notion of polychronous group will serve as an anchor point for researching breakthrough algorithms.
The main application in the proposal is related to time-frequency patterns of sonar and biosonar signals sent by animals, e.g. odontoceti, chiroptera, appear like a series of chirps that are eminently suitable to match with the activity patterns of an SNN. Especially certain echo phenomena combined with SNN modeling could help resolve the ambiguities and build features that contain information about the issuer and the target the animal chases. More generally, the study could help understand biosonar signals that are the main media for acoustic communication between mammals. The teams working for the present project propose to test and compare on biosonar signals the methods they aim to discover and develop.
If successful, the benefits could be applied to many other civilian or military research areas, for studying submarine or land sonar, or Doppler in biomedecine, everywhere where on-the-fly volatile information must be processed from sensor recordings. Speech or video recognition, as well as weather observations processing will also be used as benchmarks since we have big data in the context of ongoing PhD thesis (Affective Computing - Climate Informatics).
Ultimately the scientific issues are wide for new technologies since SNNs are highly suitable for hardware implementation. New information processing nanodevices such as memristors could lead to ultra low power neuromorphic architectures able to be used in IoT devices such as sensors, whereas todays artificial networks require data center scale computing power for their training. For understanding the strategic issues, recall that the Reservoir Computing (mainly based on SNNs) as a research topic was develop in 2007, at the same time as Deep Learning in artificial neural networks, which presently is a leading domain at the international level (see NIPS'2016). One reason for this lag could be that deep networks are technically easier to imple30 000ment and use than SNNs, but another one is that Deep Learning was mainly developed in North America, whereas SNNs are mostly studied in Europe. The success of deep neural networks has also pressed the urgency for efficient and improved spatio-temporal pattern recognition as promised by SNNs. Hence a great challenge has to be taken up by the European Union and the goal of the present proposal is exactly to build a European consortium, gathering research and development teams, to do so.