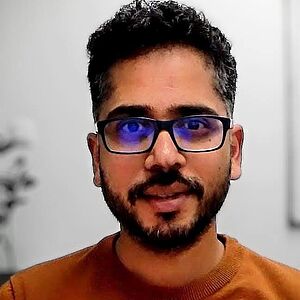
Siddharth Sivankutty
Axes de recherche
Photonics neural networks
Photonic lanterns : a novel computational platform
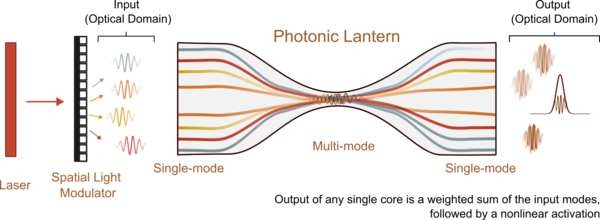
Our chosen platform for these experiments consists of photonic lanterns based on multicore optical fibers. Drawing an analogy to a single layer of a neural network, the physical cores of a multicore fiber serve as the input and output neurons of our system. Data is encoded onto either the amplitude or the polarization state of a laser beam. The weighted addition and activation function are emulated by the photonic lantern due to the inherent mode-mixing in the multimode section and nonlinear responses triggered in the single-mode sections. By engineering parameters of the lantern, such as coupling strength/geometry, nonlinearities, and recurrence, we can systematically analyze the impact of mode-mixing and higher-order nonlinearities on the network's performance. As part of an ongoing ANR project (ANR-NOLANN), we will investigate the operation of these hybrid fibers to demonstrate coherent spike generation, leveraging their rich spatio-temporal dynamics. This aligns closely with current research efforts in neuromorphic computing at IRCICA.
Frequency combs : a versatile resource for computation
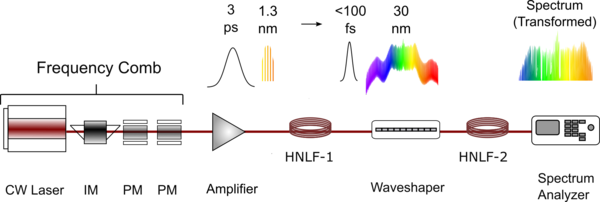
We are also exploring the possibility of implementing kernel methods in machine learning within the optical domain. In this approach, the composite kernel is inherently defined by the physical system—a highly nonlinear fiber. Leveraging the capabilities of agile frequency combs (in collaboration with Prof. Arnaud Mussot, Axis: Nonlinear fiber optics) and spectral shapers to encode data in the spectral features of the comb at high density, we aim to perform tailored nonlinear transformation of the input data directly in the optical domain. This operation enables subsequent processing using standard linear numerical methods in the digital domain. Potential applications include compression at the acquisition stage and privacy-preserving operations.
Novel imaging systems
Lensless endoscopes : Nonlinear imaging through a fiber
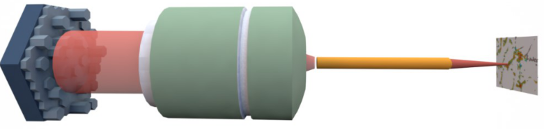
A lensless endoscope relies on optical fibers, either multimode (MMF) or multicore (MCF), to transmit images from their distal tip to the proximal end. However, mode mixing within the fibers often distorts the image. Physical approaches exploit the fact that, for coherent transport through the fibers, even with strong mode mixing, image information is not lost but merely scrambled. By adopting the transmission matrix framework, one can measure the transmission matrix of any fiber (MMF or MCF) and recover the image information. Using this powerful conceptual tool, we address the challenge of performing nonlinear imaging at the tip of an ultra-thin fiber probe. This involves delivering an ultrashort pulse through the fiber with minimal temporal broadening and focusing and scanning an intense focal spot in all three dimensions.
Interferometric lensless imaging
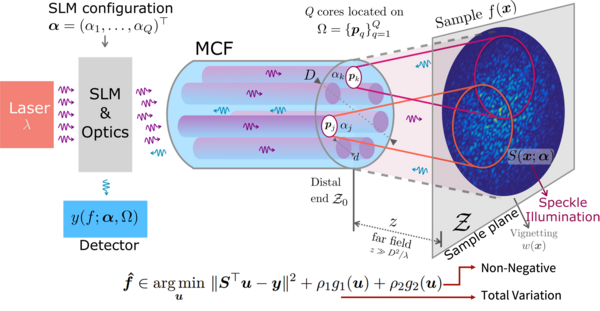
In this alternative approach, we do not seek to physically invert the transmission matrix but rather computationally retrieve the image. This approach leverages both an interferometric image formation model and the bending-invariance property of our multicore fibers. Posing the retrieval of images as an inverse problem involving rank-one projections of the object spatial frequencies, moves the complexity from the instrumentation to the numerical reconstruction. Hence, this technique has the potential to alleviate several technical and cost requirements for high-speed imaging via an ultra-thin probe.